Data Fusion of GNSS with LEO Satellites as Alternate-PNT Systems Including Anomaly Detection
The objective of this proposed project is a novel software-defined, resilient, network-based Position, Navigation, and Timing (PNT) system that has the potential to provide robust PNT for single-weapon and network-enabled weapon systems of interest to AFRL/RWWN. This proposed technology efficiently utilizes network signals from Low Earth Orbit (LEO) satellites (LEO PNT), Time Difference Of Arrival (TDOA), and Kalman filtering methods to provide accurate and precise PNT. Note that there is no need to collaborate with, or communicate via, the LEO satellites themselves. Rather, the proposed technology uses the LEO Satellites as RF Signals Of Opportunity (SOOP). Reference stations (stationary or mobile) at known locations measure TOA data of the LEO satellites that they can opportunistically receive. These TOA data are securely streamed to Command-and-Control (C&C) servers located in-theatre or secured in the cloud. The C&C servers compute position, velocity, altitude (PVA) for all of the LEO satellites that are in-theatre and stream the resulting LEO PVA and TOA to the in-theatre assets e.g., Aircraft, Drones, Munitions, and Mounted or Dismounted warfighters. Given the PVA and TOA, this technology enables assets to navigate to waypoints and targets to sub-meter accuracy using alt-PNT as a robust alternative to the easily spoofable and jammable Global Navigation Satellite Systems (GNSS). Proposed alt-PNT algorithms are modular to facilitate integration with existing RF network interfaces or use advanced low-probability of detection/interference (LPOD/I) Ultra-Wide-Band (UWB) network transceivers, and very importantly, their implementation is intended on COTS Software Defined Radios (SDR). All PVA and TOA data are recorded at the C&C servers for forensic analysis and algorithm testing and improvement.
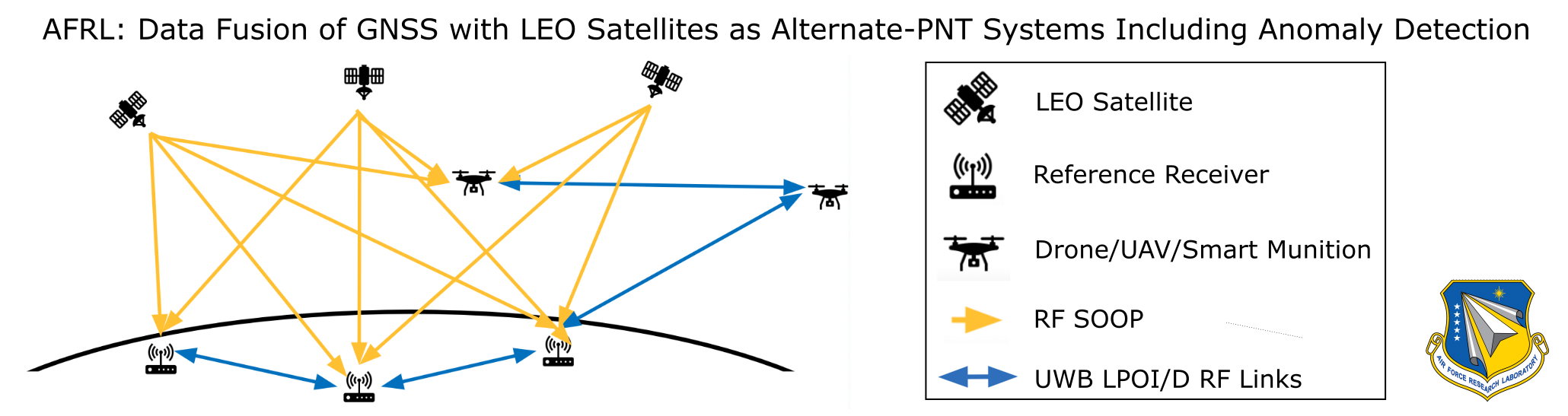