MultiHyRL: Robust Hybrid RL for Obstacle Avoidance against Adversarial Attacks on the Observation Space
The objective of this work is to generate new fundamental science for hybrid dynamical systems that enables systematic design of algorithms using reinforcement learning (RL) tech- niques that are hybrid. Hybrid systems are dynamical systems with intertwined continuous and discrete behavior. Hybrid controllers are algorithms that involve logic variables, timers, memory states, along with the associated decision-making logic. The combination of such mixed behavior, both in the system to control and in the algorithms, is embodied in key future engineering systems. The future autonomous systems will have variables that change continuously according to physics laws, exhibit jumps due to controlled switches, replanning of maneuvers, on-the-fly redesign, and failures, while the control algorithms require logic to adapt to such abrupt changes. Hybrid behavior also emerges in such engineering systems due to their complexity. In fact, communication events, abrupt changes in connectivity, and the cyber-physical interaction between vehicles, humans, robots, their environment, and com- munication networks lead to impulsive behavior that interacts with physics and computing. In this project, we propose to develop novel hybrid reinforcement learning control algorithms that can be validated in experimental data-driven testbeds. The proposed combination of hybrid control and learning informed by real-time data exploits – in a holistic manner – key robust stabilization capabilities of hybrid feedback control and learning capabilities of RL for autonomous systems of interest to AFRL that are part of the broad mission of the DoD.
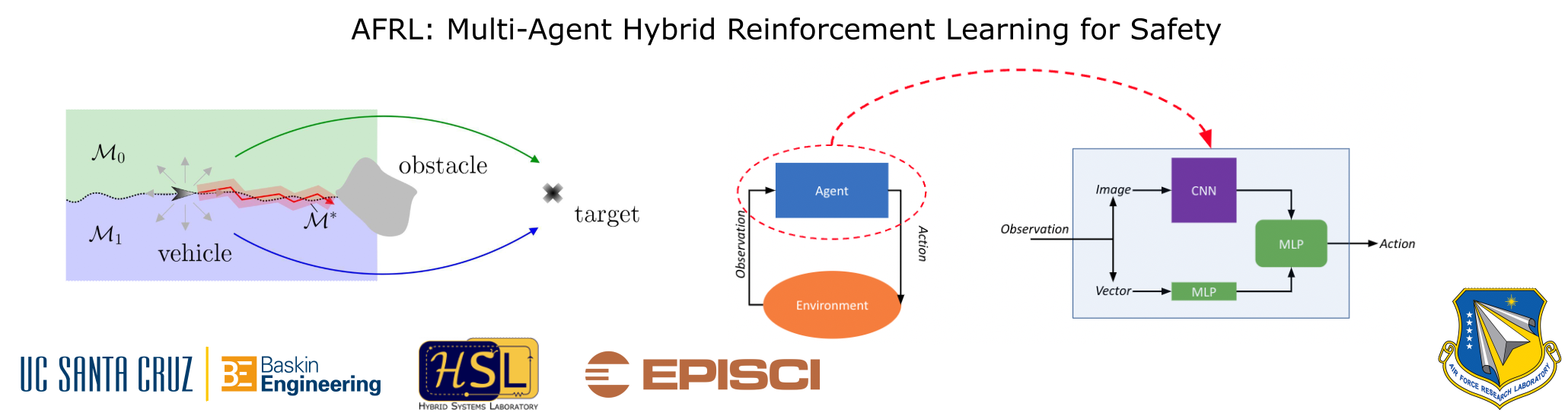