Systematic Tools for Satisfying Temporal Logic Specifications in Hybrid Dynamical Systems: A Control Theoretical Approach
This proposal aims to develop tools that permit guaranteeing high-level specifications for solutions to hybrid dynamical systems that neither require discretization of the dynamics or of the state space, nor the computation of the solutions themselves. Our research is motivated by the fact that Air Force combat systems require autonomous systems that are agile, reliable, and resilient to meet stringent requirements of its missions. Unfortunately, the unavoidable combination of the physics, the networks, and the algorithms in such systems leads to hybrid system models, which makes the systematic design of algorithms very challenging. The unavoidable presence of obstacles, adversarial elements, and uncertainty in the environment further adds to the complexity and requires design tools that neither discretize the state or the dynamics while guaranteeing given high-level specifications, robustly. While discretization may aid computations in some cases, discretization of the hybrid system models emerging in Air Force missions may not permit assurance that the desired specifications are actually satisfied.
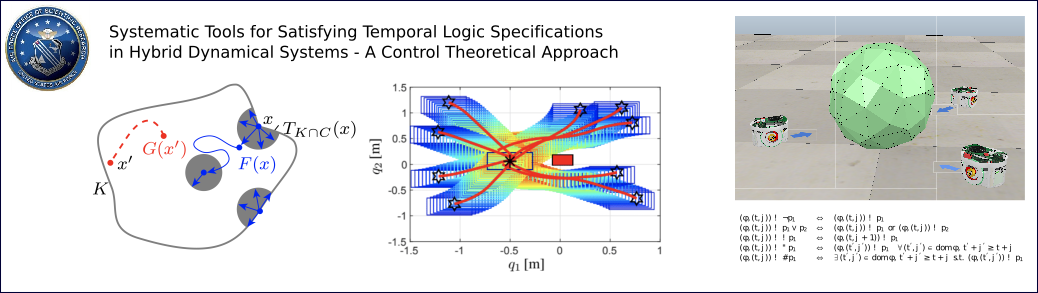